High-fidelity simulations of turbomachinery applications
The long-term objective of this high-fidelity simulation program is to generate the fundamental understanding and low-order design tools required to develop the next generation of efficient and environmentally friendly turbines for power generation and air transport. Overall, our research team is focused on shedding light on the most significant loss generation mechanisms inside of gas turbines, how to further improve turbine cooling and identifying and addressing noise generation mechanisms of jets and wind turbine blades. In 2018, high-fidelity simulations have been conducted of fluid flows occurring in turbomachinery applications with an in-house code specifically designed and optimised to exploit the performance of supercomputers. We have conducted simulations at realistic engine conditions, giving us unprecedented understanding of the physical phenomena occurring in these flows. The data is used to help assess the current low-order models that are employed in an industrial environment. The data also serves as gold-standard database for developing new and improved models based on machine-learning approaches
Area of science
Applied Science, Chemistry
Systems used
Magnus
Applications used
The Challenge
The long-term objective of this high-fidelity simulation program is to generate the fundamental understanding and low-order design tools required to develop the next generation of efficient and environmentally friendly turbines for power generation and air transport. Overall, our research team is focused on shedding light on the most significant loss generation mechanisms inside of gas turbines, how to further improve turbine cooling and identifying and addressing noise generation mechanisms of jets and wind turbine blades. In 2018, high-fidelity simulations have been conducted of fluid flows occurring in turbomachinery applications with an in-house code specifically designed and optimised to exploit the performance of supercomputers. We have conducted simulations at realistic engine conditions, giving us unprecedented understanding of the physical phenomena occurring in these flows. The data is used to help assess the current low-order models that are employed in an industrial environment. The data also serves as gold-standard database for developing new and improved models based on machine-learning approaches.
The Solution
Australia relies heavily on gas turbines (GTs) for propulsion- and power-generation. Given the very large installed base nationally, and worldwide, any GT efficiency increase has significant potential to reduce fuel burn and environmental impact. One of the keys to improving the operating efficiency of GTs is the ability to understand and predict the detailed flow behaviour inside of the engine. The challenge is that the flow inside an engine is mostly turbulent, and turbulence is a highly chaotic process that is extremely hard to predict. Today’s design of gas turbines is driven largely by computational fluid dynamic (CFD) modelling. However, some of these turbulence models are really only accurate in “known” situations, where the flow behaviour is well recognised and the models have already been calibrated. If off-design conditions or more radical novel concepts are to be considered, these models cannot be trusted. In addition the accurate prediction of the turbulent heat transfer is a key design consideration for ensuring survival of key turbine components and hence reliability of turbines. This research also aims to improve heat transfer models that are even less reliable and mature than current turbulence models.
The Outcome
Model-free direct numerical simulations of turbulent flow and heat transfer with relevance to gas turbines are only possible on very large supercomputers. The access to Magnus has allowed us to conduct a range of high-fidelity simulations that would not have been possible on local resources. Various cases have been studied, from looking at loss mechanisms in low-pressure turbines, in particular the losses generated by endwall effects, trailing-edge slots for turbine cooling, to first-principle-based simulations of turbulent combustion with simple chemistry, and ways of reducing noise from blades in engines.
List of Publications
[1] Pichler, R., Zhao, Y., Sandberg, R.D., Michelassi, V., Pacciani, R., Macroncini, M., Arnone, A., 2018 “LES and RANS Analysis of the End-Wall Flow in a Linear LPT Cascade: Part I — Flow and Secondary Vorticity Fields Under Varying Inlet Condition”, ASME IGTI, GT2018-76233.
[2] Macroncini, M., Pacciani, R., Arnone, A., Michelassi, V., Pichler, R., Zhao, Y., Sandberg, R.D, 2018 “LES and RANS Analysis of the End-Wall Flow in a Linear LPT Cascade With Variable Inlet Conditions: Part II — Loss Generation”, ASME IGTI, GT2018-76450.
[3] Marconcini, M., Pacciani, R., Arnone, A., Michelassi, V., Pichler, R., Zhao, Y., Sandberg R.D., 2018 “LES and RANS Analysis of the End-Wall Flow in a Linear LPT Cascade with Variable Inlet Conditions, Part II: Loss Generation”, accepted for J. Turbomachinery 20/08/2018
[4] Akolekar, H. D., Weatheritt, J., Hutchins, N., Sandberg R.D., Laskowski, G., Michelassi, V., 2018 “Development and Use of Machine-Learnt Algebraic Reynolds Stress Models for Enhanced Prediction of Wake Mixing in LPTs”, ASME IGTI, GT2018-75447.
[5] Akolekar, H.D., Weatheritt, J., Hutchins, N., Sandberg R.D., Laskowski, G., Michelassi, V., 2018 “Development and Use of Machine-Learnt Algebraic Reynolds Stress Models for Enhanced Prediction of Wake Mixing in LPTs”, accepted for J. Turbomachinery 01/10/2018
[6] Lengani, D., Simoni, D., Pichler, R., Sandberg, R.D., Michelassi, V., Bertini, F., 2018 “Identification and quantification of losses in a LPT cascade by POD applied to LES data”, International Journal of Heat and Fluid Flow, 70(4), 28-40.
[7] Lengani, D., Simoni, D., Pichler, R., Sandberg, R.D., Michelassi, V., Bertini, F., 2018 “On the Identification and Decomposition of the Unsteady Losses in a Turbine Cascade”, ASME IGTI, GT2018-75724.
[8] Lengani, D., Simoni, D., Pichler, R., Sandberg R.D., Michelassi, V, Bertini, F., 2018 “On the Identification and Decomposition of the Unsteady Losses in a Turbine Cascade”, accepted for Journal of Turbomachinery 20/08/2018
[9] Sandberg R.D., Tan, R., Weatheritt, J., Ooi, A., Haghiri, A., Michelassi, V., Laskowski, G., 2018 “Applying Machine Learnt Explicit Algebraic Stress and Scalar Flux Models to a Fundamental Trailing Edge Slot”, ASME IGTI, GT2018-75444.
[10] Sandberg R.D., Tan, R., Weatheritt, J., Ooi, A., Haghiri, A., Michelassi, V., Laskowski, G., 2018 “Applying Machine Learnt Explicit Algebraic Stress and Scalar Flux Models to a Fundamental Trailing Edge Slot”, ASME J. Turbomach., 140(10), 101008.
[11] Ma, D. Talei, M., Sandberg, R.D., 2018, “DNS of a Turbulent Premixed Jet Flame with Fully Developed Turbulent Inlet,” 21st Australasian Fluid Mechanics Conference, Dec 10-13, 2018, Adelaide, Australia
[12] Wu, H., Sanjose, M., Moreau, S., Sandberg, R.D. (2018) “Direct Numerical Simulation of the Self-Noise Radiated by the Installed Controlled-Diffusion Airfoil at Transitional Reynolds Number” AIAA/CEAS Aeroacoustics Conference 2018-3797, 1-17
Figure 1. LPT_endwall.png: Secondary vortical structures of turbine endwall flows identified by streamlines
Figure 2. Turb_Combust.png: Iso-surfaces of second eigenvalue of velocity gradient tensor (green) and reactant mass fraction (red) for a turbulent flame
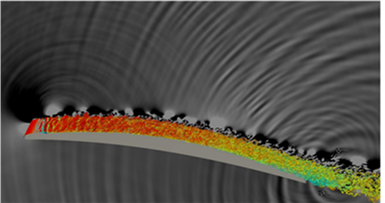
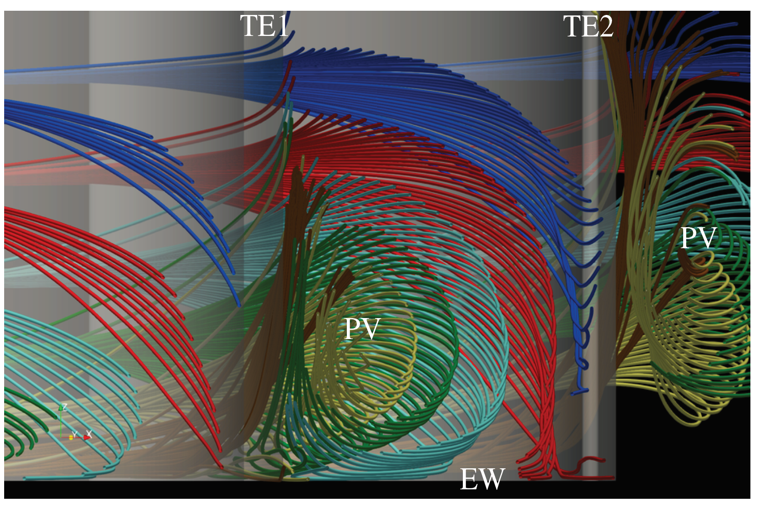